Video super-resolution (VSR) refers to improve the viewing experience on picture quality with respect to the video scenarios while effectively reducing the production and transmission costs of the video by analyzing the pictures and scenarios through deep learning technology and processing them by means of de-noising, deblurring, sharpening, de-shaking and other picture quality enhancements, etc.Recently, based on the technology accumulation in video coding and decoding technology, algorithms, compilation optimization, etc., JD Cloud Video Cloud has launched the super-resolution SDK products for mobile terminals (including mainstream terminals such as Android and IOS), and practically applied them in the JD Mall APP. After the verification by long-term data iteration and data monitoring, enabled by super resolution, the average play time of users is increased by 80% and the cost of traffic bandwidth is reduced by 30%, which effectively improves user experience and GMV conversion.02 Technological Implementation
The existing image and video super resolution technologies can be divided into two major categories, namely SISR and VSR. The former realizes one-to-one super resolution based on single-frame image, while the latter realizes many-to-one super resolution based on time-domain attributes of multiple video frames. At present, JD Cloud Video Cloud has a complete image and video super-resolution solution, which has been implemented in live video and VOD scenarios. The following describes in detail the technical implementation of super resolution-enabled businesses for real-time live streaming scenarios.Currently, SISR mainly involves nine methods, including linear networks, redisual networks, recursive networks, and GAN models. Due to the high requirements for real-time performance, computational complexity, stability, etc. in live streaming business scenario, we mainly make engineering improvements, optimize the video processing process, and specifically deal with burrs, block effects, text blurring, video jitter, etc., to improve the video viewing experience by adopting ESPCN (the algorithm of linear networks) based on video ROI characteristics. After ESPCN moves the up-sampling to the convolution operation, it performs image feature extraction and nonlinear feature mapping at the LR layer first, and finally realizes up-sampling by subpixel convolution. Compared with traditional SRCNN, it saves much network computation. In this implementation, videos are segmented into multiple slices based on ROI in accordance with video features, and segmented into long, rectangular, square and other regular coding strips according to macroblock integers, and are divided into ROI area and non-ROI area.The ROI area is based on ESPCN super resolution. In the non-ROI area, the traditional upsampling algorithm Bicubic is adopted to finally splice the YUV/RGB full-frame image. In this way the convolution operation based on the complexity of the full-size image and the largest amount of computation can be segmented to focus on the region-based image convolution, greatly improving the calculation complexity, and realizing 1080p~4K super resolution in real time and with lower performance. The processing flow of single-frame image is shown in the figure below:Human eyes are very sensitive to brightness signals, so it is necessary to reconstruct brightness signals by super resolution. High-resolution chroma signals are reconstructed by traditional difference method.
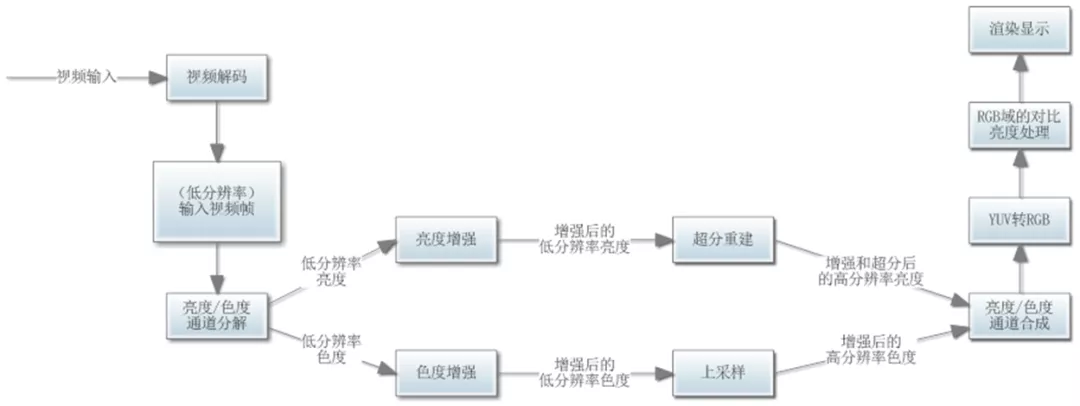
Figure 3 Processing ProcessAccording to the processing process as shown in the figure, the video is decoded to obtain low-resolution video images first, and then the brightness and chroma signals are decomposed and enhanced respectively. High-resolution chroma and brightness signals are obtained by performing super-resolution reconstruction on the enhanced brightness signals and upsampling the enhanced chroma signals. Finally, the brightness and chroma signals are combined, and the YUV is converted to RGB to obtain the final high-resolution video images.
04 Technology Application
The actual application effect of JD Cloud Video Cloud’s super-resolution SDK products for mobile terminals in JD Mall APP is as follows:
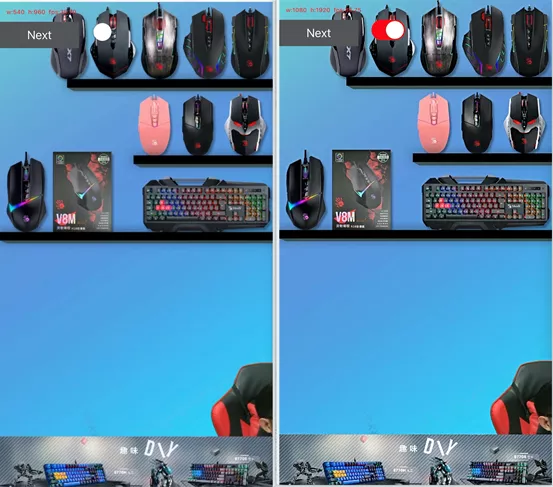
Comparison of Subjective Effects of Super Resolution
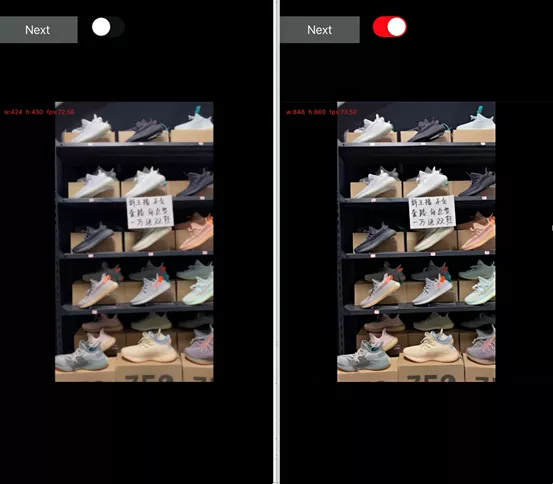
Comparison of Subjective Effects of Super Resolution
Super Resolution / Traditional Method Vs. Source
|
Video | PSNR | VMAF | SSIM |
540x960 | 33.43/30.21 | 92.325325/78.163467 | 0.917754/0.832233 |
424x430 | 32.55/30.43 | 93.455247/79.474218 | 0.924242/0.896367 |
Comparison of Objective Evaluation Data
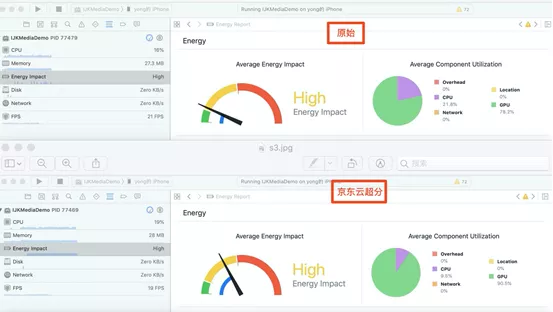
Comparison of Power Consumption Performance
As a leading video cloud service provider in China, JD Cloud Video Cloud provides a range of products covering live video, video-on-demand (VOD), real-time audio and video platforms, full-end SDK, and have integrated the whole process of video acquisition, editing, playing, storage, management, review, and distribution. Based on the technological breakthroughs in video coding, algorithm optimization, audio analysis and processing, real-time audio and video, etc., the products enjoy relatively leading technical advantages in JD super-definition transcoding, comfortable audio, real-time audio and video communication, ultra-low-latency live streaming and other functions, and can provide customers with full scenario-based end-to-end video solutions.